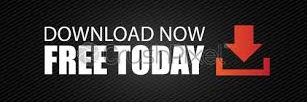
Think of semantic annotations as a sort of highly structured digital marginalia ( notes made in the margins of a book or other document), usually invisible in the human-readable part of the content. This approach is implemented in Ontotext Platform.ĭo you want to learn how Ontotext uses semantic annotation to enrich your content?Ĭreate Smart Content with Machine-Processable Marginalia This way documents and annotations become first class citizens of the knowledge graph and can be indexed and queried alongside the other type of data there: ontologies, schemas, reference and master data. One modelling approach, which enables a broad range of analytics, is to store the annotations as individual objects, which refer to the document, which is also a node in the graph. Semantic metadata can be stored in a knowledge graph, rather than embedded in a document. Typically, such metadata is represented as a set of tags or annotations that enrich the document, or specific fragments of it, with identifiers of concepts. These references link the content to the formal descriptions of these concepts in a knowledge graph. The result of the semantic annotation process is metadata that describes the document via references to concepts and entities mentioned in the text or relevant to it. Semantically tagged documents are easier to find, interpret, combine and reuse. Unlike classic text annotations, which are for the reader’s reference, semantic annotations can also be used by machines. Semantic annotation or tagging is the process of attaching to a text document or other unstructured content, metadata about concepts (e.g., people, places, organizations, products or topics) relevant to it.
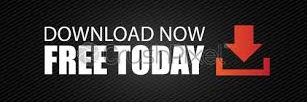